AI Defect Detection Solution
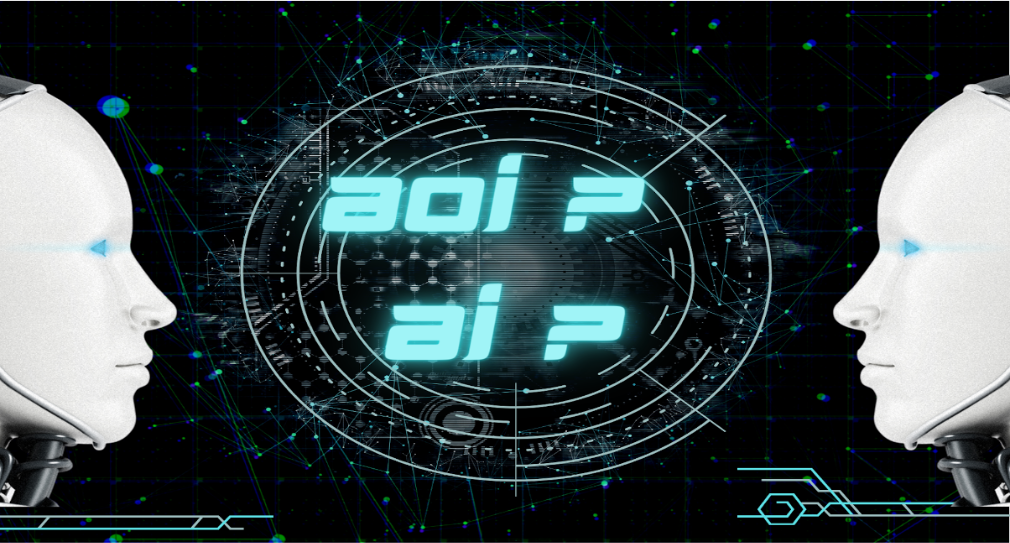
The rapid development of technology has made AI (Artificial intelligence) a crucial topic across various industries. AI applications are extensive, ranging from preventive maintenance and supply chain optimization to visual inspection, with visual inspection being one of the most widely utilized. AI visual defect detection is a method that employs artificial intelligence technology to automatically detect defects or flaws in products or processes. This detection method is based on technologies such as machine learning and deep learning, utilizing large amounts of visual data to train and optimize models for recognizing and categorizing various types of defects.
As GPU capabilities have become increasingly powerful and with the rise of AI research, many industries are actively embracing digital transformation, and the manufacturing industry stands out as one of the most visibly transformed sectors. In manufacturing, AI defect detection can be applied to various fields, including electronic components, automotive parts, semiconductors, and food packaging. The primary purpose of applying AI in these areas is to enhance the efficiency of inspections and the accuracy of products, while simultaneously reducing human errors and defects during production, ensuring that the product quality aligns with customer requirements.
AI vs. AOI
Currently, most manufacturing industries still rely on AOI (Automated Optical Inspection) for product inspection. The detection principle of AOI involves logic judgment based on preset parameters, significantly improving upon the traditional practice of using human labor and optical instruments for inspections. Despite decades of refinement, AOI technology has become highly mature and can effectively replace manual visual inspections. However, with changes in the consumer market leading to higher proportions of small-batch and diversified production in the manufacturing industry, the variety of inspection conditions has increased. Relying solely on rule-based standard defect recognition encounters bottlenecks, including difficulties in precisely defining irregular defects or identifying unexpected ones. These issues can result in process waste, and AOI faces challenges in overcoming them, requiring more flexible mechanisms to assist machines in making judgments.
The maturity of AI technology presents an opportunity to address the limitations of AOI, improving detection efficiency and effectively reducing false positives. During the initial stages of AI implementation, when there is a limited amount of data, ensuring real-world accuracy can be challenging. At this point, AOI can assist in rapidly collecting data, shortening model convergence time. Consequently, the combination of "AOI+AI" has gradually become a mainstream method to enhance detection accuracy.
Although AOI and AI are often compared, they are not substitutes but complementary tools. Based on their different principles of use, they have different application scenarios, and choosing the most effective method according to specific needs can greatly assist customers in achieving optimal results.
Benefits of AI Visual Inspection
In recent years, end customers have become increasingly demanding in their expectations for product quality. To meet these expectations, many enterprises are now requiring manufacturers to utilize AI technology to ensure the quality of their products. AI achieves this by feeding on extensive datasets and continuously engaging in deep learning to identify complex defects under various inspection conditions. The accuracy of AI improves with the expansion of its image library, and it demonstrates a higher tolerance for environmental variations, enabling quick adaptation to changes. This capability enhances quality control, and when compared to AOI, AI contributes to improving production efficiency, reducing production costs, and ensuring product yield.
AI Visual Defect Detection is utilized in the following ways:
1. Accelerated the speed of detection
→ Utilizing a mature AI detection model with comprehensive optical hardware enhances image recognition precision, elevating software reliability and recognition speed.
2. Improvement of detection accuracy
→ Continuously collecting defect samples and completing data preprocessing, through multiple rounds of model training, better aligns with the inspection requirements of the production line.
3. Recognize complex defects
→ Accurately annotating features and scopes of defects enables the AI model to autonomously learn, facilitating precise identification of a diverse range of defect types.
4. Inspection Data Digitization
→ Collecting detection results and regularly generating reports aids in reviewing process issues and implementing corrections for continuous improvement.
"How to maximize the performance of AI technology on the production line?" has always been an important issue for enterprises in introducing AI. Spingence provides a mature and complete process of introducing AI visual defect detection solutions, with easy-to-use interfaces, flexible and iterative models, and open ecosystems, to help AI technology bring unlimited value to the production line!